Data Superpowers: Skew Correction
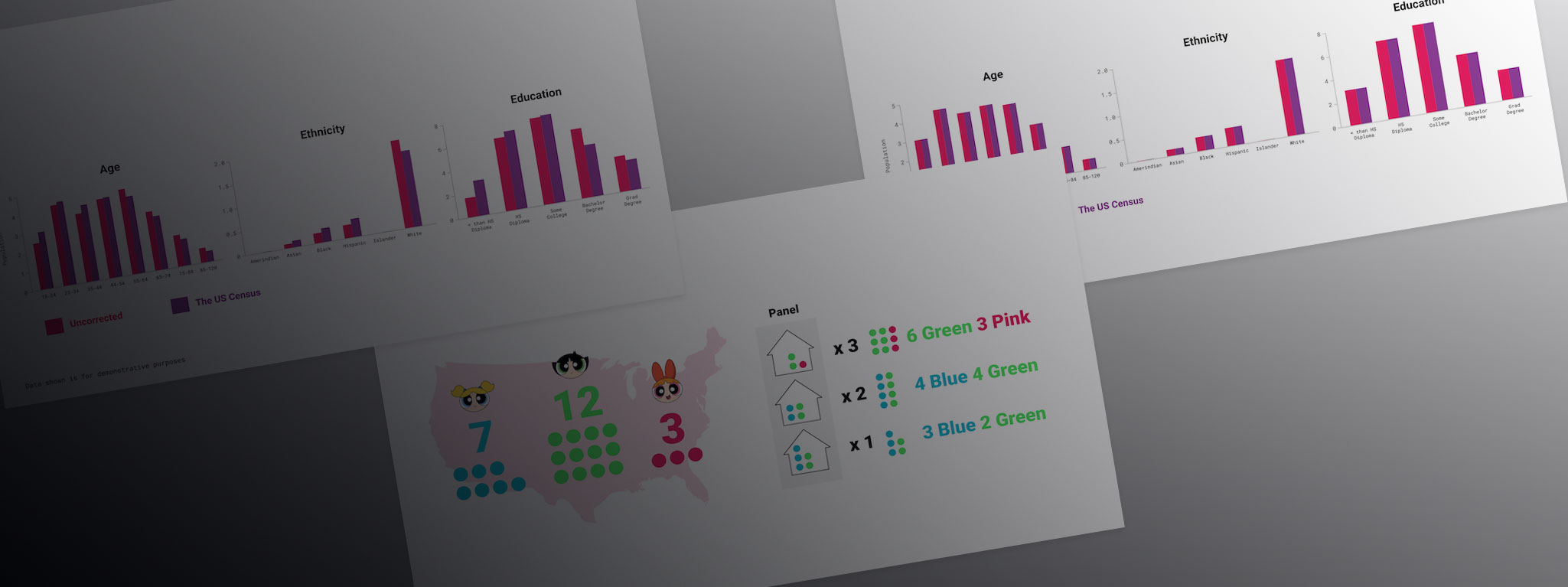
At VideoAmp, we deal with a lot of complex topics, which is sort of par for the course working in adtech. In an effort to develop solutions that add value to our client’s media spend, our team of data scientists focus on implementing technical changes and developing algorithms that help support this goal.
One of these topics is skew correction. It used to be very easy for advertisers to target an audience and know they’d seen a specific creative based on their media buy. With the proliferation of devices, and now the availability to measure ad effectiveness across digital, TV’s are no longer an accurate representation of the U.S., and even less so when you’re looking for specific audiences.
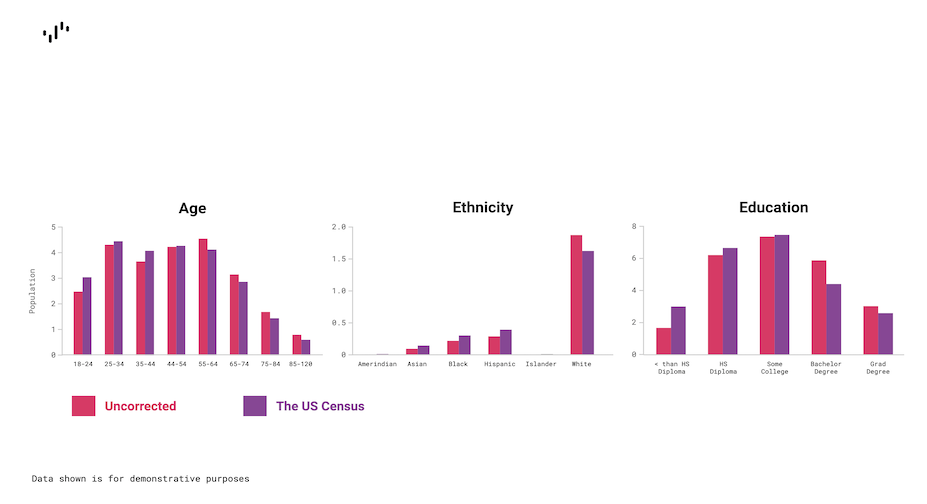
At VideoAmp, we use our commingled panel (ACR + STB) to model TV viewership behavior on the national scale. The population of households in the VideoAmp panel is a biased sample of national households. This bias must be corrected for; we call this process skew correction. An example of this would be looking at a skewed representation of an audience that shows demographics to be older than is actually correct. By not assigning weights to households to account for different demographics within that household, significant data inaccuracies and, ultimately, poor decisions, are made by advertisers. When tech providers share this panel information, without making any corrections, they’re essentially telling advertisers to push media dollars towards programming that suits this older audience- without knowing if they’re actually the one’s watching.
So, how do we do it? Our process prevents these biases from happening by assigning each household a weight, normalizing the sample’s demographic representation to match the true demographics of the US. If you’re confused, you’re not alone.
Imagine that the country is made up entirely of Powerpuff Girls (a late 90’s fan favorite for many of us at VideoAmp), represented by the chart below; 7 copies of Bubbles (blue), 12 copies of Buttercup (green), and 3 copies of Blossom (pink). If your panel consists of only three households with the members shown on the right, your data is heavily skewed against Blossom, representing only 8% of the panel, as opposed to a more accurate 14% of the country.
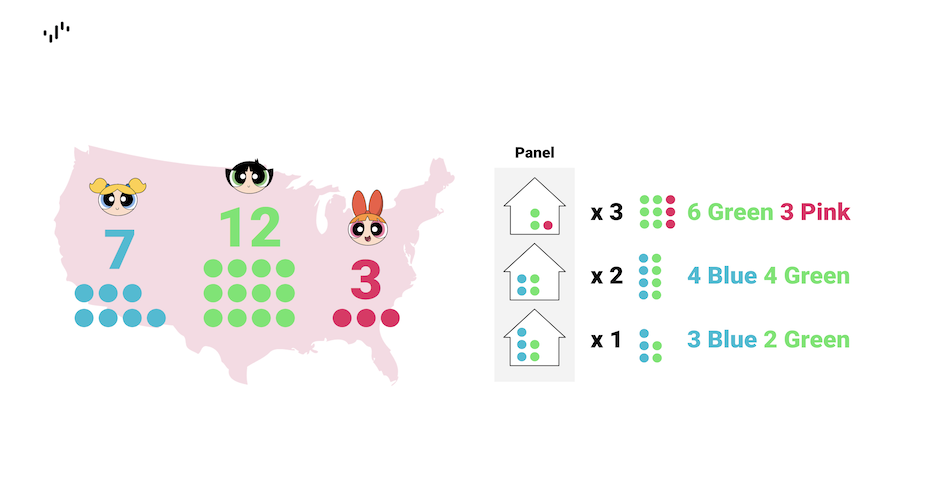
To solve for this bias, we treat each household as a piece to the overall national puzzle. To complete the puzzle, we need to figure out how many copies of each household piece are needed to build up the true numbers of the total Powerpuff Girls in the country. We can do this exactly with the following breakdown: 3 copies of Household 1, 2 copies of Household 2, and 1 copy of Household 3. The required number of copies for each household is exactly that household’s weight.
Sadly, things are slightly more complicated in reality. In our datasets, we are simultaneously correcting for sampling bias in over two hundred demographic categories using both the U.S. Census and VideoAmp’s own intab panel as ground truths. To do this, we first translate the simplified example using Powerpuff Girls into a regularized matrix equation. Next, we leverage a proprietary learning algorithm to solve for more than 10 million weights corresponding to each household in our commingled dataset. Through this process, we make our panel representation more accurately modeled and skew-corrected to the U.S. census and typical TV viewing behavior.
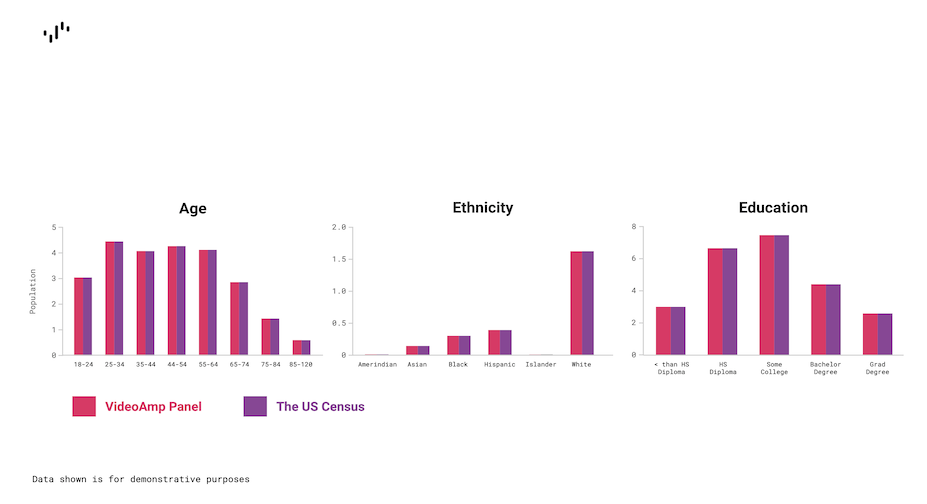
Our goal is to enable advertisers to make the smartest, data-driven business decisions in regards to their media investment. In order to do this, you need accurate and reliable weights, which come with a bulletproof skew correction methodology. With every improvement to our methodology, and as we continue developing new ways to move the needle forward, we are paving the way for new features involving weights down the road.
Skew correction for customizable ground truths beyond the standard US census, cross-screen weights, and on-demand weights for custom time ranges and audiences are just a few of the groundbreaking developments we continue to work towards.